From 2016, various results have been found and published. Following are some examples:
Sequence to Sequence Weather Forecasting with Long Short-Term Memory Recurrent Neural Networks
This work is based on a deep neural network architecture, and use it in time series weather prediction. It uses multi stacked LSTMs to map sequences of weather values of the same length. The goal is to produce two types of models per city (for 9 cities in Morocco) to forecast 24 and 72 hours worth of weather data (Temperature, Humidity and Wind Speed). Approximately 15 years (2000-2015) of hourly meteorological data was used to train the model. The results show that LSTM based neural networks are competitive with the traditional methods and can be considered as a better alternative to forecast general weather conditions.
A Java Application to Process Environmental and Pollution Datasets
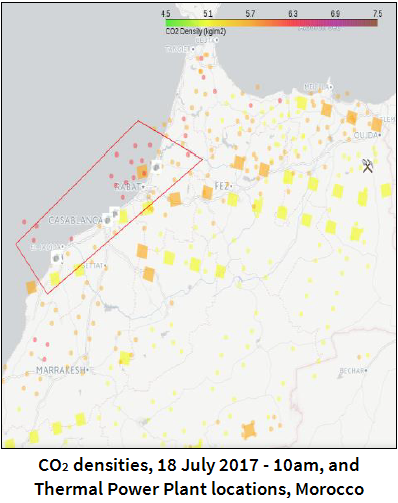
A Java-based application was developed to collect, process and visualize environmental and pollution datasets, acquired from the Mediterranean Dialog earth Observatory (MDEO) platform. This application amasses data of Morocco area from EUMETSAT satellites, decompresses, filters and classifies the received data. The processed data are used to build an interactive environmental real-time map. This should help finding out potential correlations between pollutants and emitting sources.
MetOp Satellites Data Processing for Air Pollution MonitorinG
This work is about a data processing system based on an architecture comprised of multiple stacked layers of computational processes that transforms Raw Binary Pollution Data coming directly from Two EUMETSAT MetOp satellites to the Lab servers, into ready to interpret and visualise continuous data stream in near real time using techniques varying from task automation, data preprocessing and data analysis to machine learning using feedforward artificial neural networks. The proposed system handles the acquisition, cleaning, processing, normalizing, and predicting of Pollution Data in the area of interest of Morocco.
An extract-transform-load software for satellite Big Data Ingestion
This work aims to prove that satellite data are big data. Accordingly, we propose a software to automatically extract, transform and load datasets, for satellite pre-processing. We focused on the ingestion layer, which will enable an efficient remote sensing big data integration. As result, the developed software layer receives data continuously, analyzes them and removes almost 86% of the unused files. Also, this layer eliminates approximately 20% of erroneous datasets. Thanks to the proposed approach, we successfully reduced the storage space usage, enhanced the remote sensing data accuracy, and integrated preprocessed datasets into a Hadoop distributed file system.
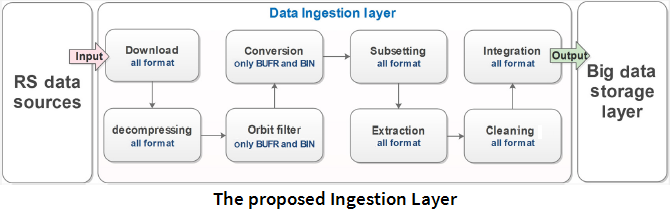